About AttentioNet
Understanding student attention is crucial for uncovering their goals, intentions, and interests, which is invaluable across various research fields, from psychology to interactive systems. Traditional methods for classifying attention often fail to capture its complex nature. To address this gap, we introduce the AttentioNet dataset, which utilises Electroencephalography (EEG) signals to classify attention into five states: selective, sustained, divided, alternating, and relaxed. The AttentioNet dataset was collected from 20 subjects who performed standard neuropsychological tasks while EEG data was collected. Each participant completed a computerised experiment, approximately 14 minutes long, involving four established neuropsychological tasks designed to stimulate different types of attention: selective, divided, sustained, and alternating. All participants self-reported not having colour blindness, a history of neurological disease, or hearing disorder; they had normal or corrected-to-normal vision. EEG data was recorded using the Emotiv EPOC+ system and Emotiv PRO software. This system enabled the wireless acquisition of raw EEG signals via Bluetooth at a high sampling rate of 128 Hz from 14 electrodes strategically positioned on the scalp. The annotations include reaction times, accuracy, and self-reported distraction levels, providing clear indicators of attention performance.
Paper Link : AttentioNet: Monitoring Student Attention Type in Learning with EEG-Based Measurement System
Data Collection Pipeline
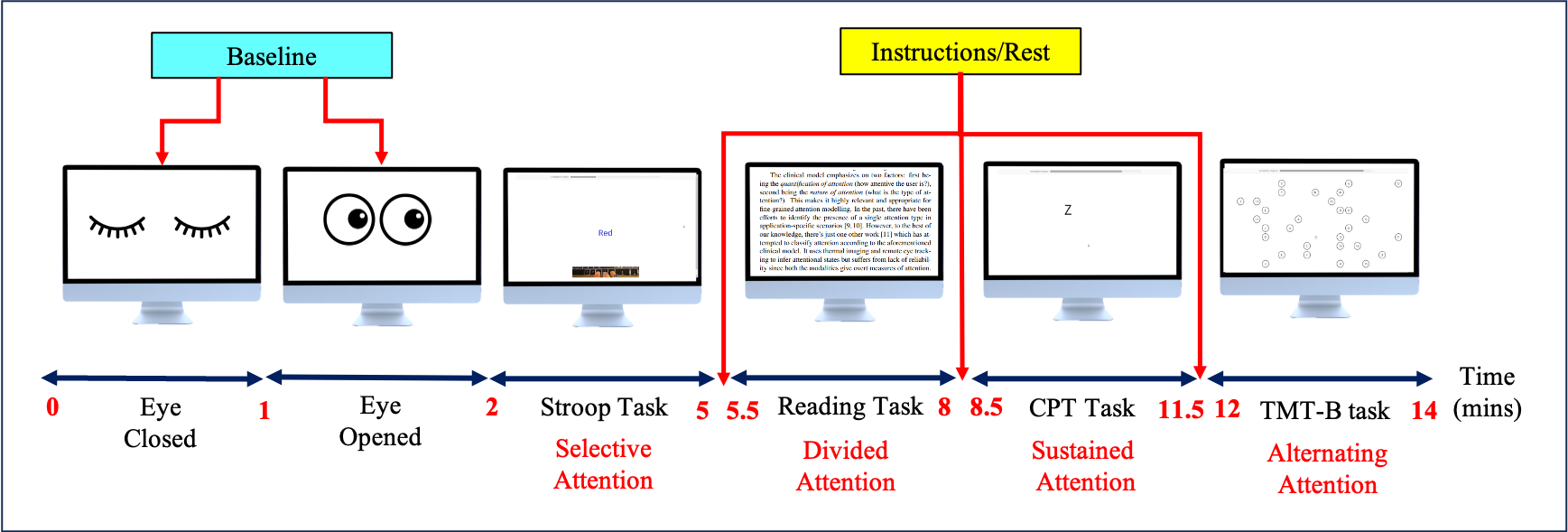
Download Instructions
Please follow these steps to access the dataset:
1. Download and print the End User License Agreement (EULA).
2. Sign the EULA and scan the signed copy.
3. Fill out the form and upload the scanned EULA using the form.
Once we receive the signed EULA, we will provide you with the link to the dataset.
Acknowledgements
We sincerely thank the participants who generously dedicated their time and effort to this study. Your contributions in providing EEG signal data and neuropsychological test scores were essential to developing the AttentioNet dataset. We deeply appreciate your patience and willingness to support this research.